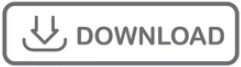
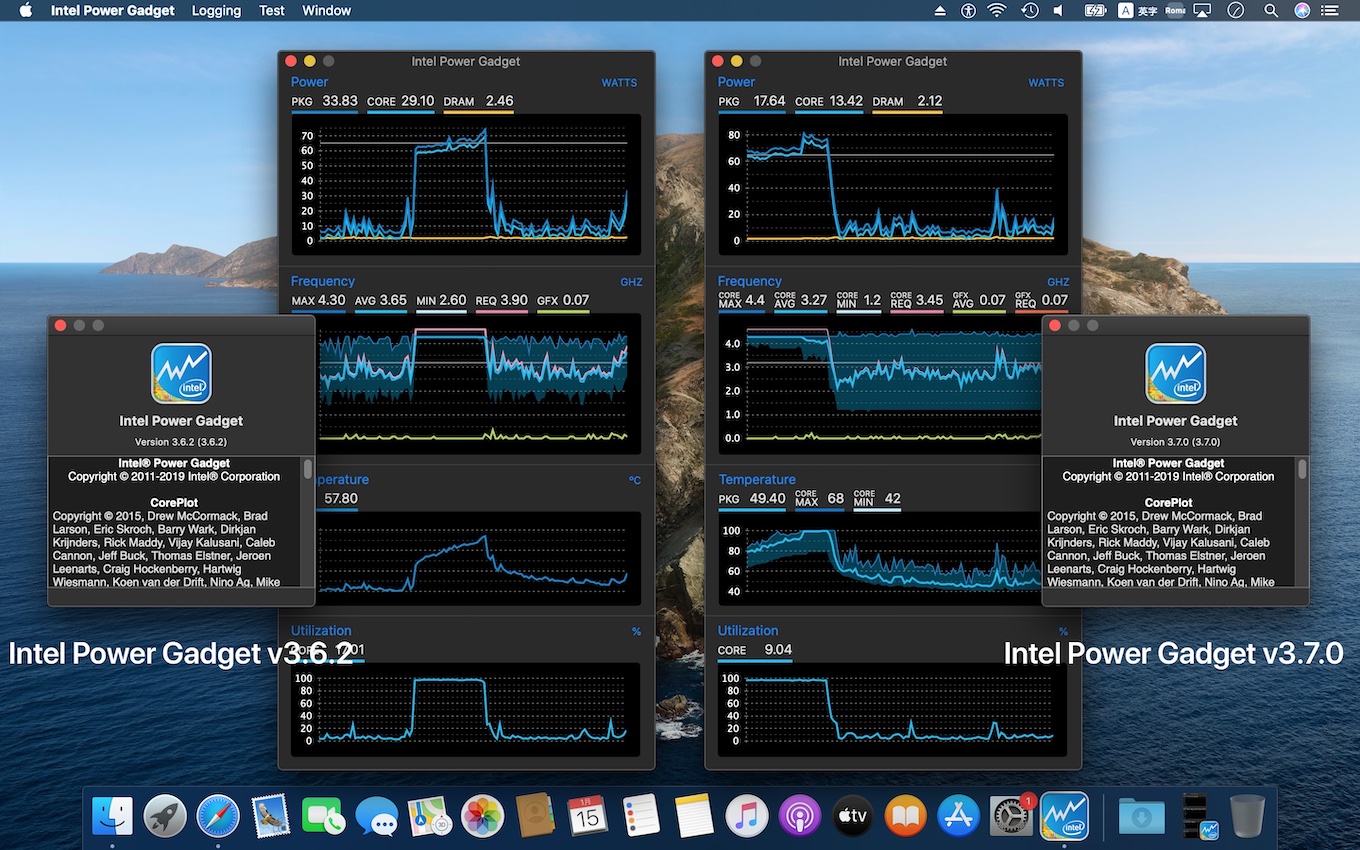
The growth of model size has been well reflected in neural networks (NNs) over the last decade, which are considered as the main ML algorithms implemented during this period.Īn optimal ML model should achieve balanced predictive performance and energy efficiency. Second, to achieve high predictive performance, the computation and memory requirements of ML models have increased. First, energy constraints constitute a major issue when ML is deployed into battery-powered medical devices. The increasing focus on inference energy can primarily be attributed to two reasons. Machine learning (ML) methods have been successfully employed in various medical fields, and energy consumption during ML inference has been attracting increasing attention.

In all experiments, the XGB algorithm exhibited the best performance in terms of accuracy, run time, and energy efficiency. Compared with a five-hidden-layer NN, the XGB and LR algorithms achieved 16%-24% and 9%-13% lower power consumption levels for the mass spectrometry and urinalysis data sets, respectively. In terms of energy efficiency, the XGB algorithm exhibited the lowest power consumption for the mass spectrometry data set (9.42 Watts) and the LR algorithm exhibited the lowest power consumption for the urinalysis data set (9.98 Watts). Compared with the RF algorithm, the XGB and LR algorithms exhibited a 45% and 53%-60% reduction in inference time for the mass spectrometry and urinalysis data sets, respectively. The XGB and LR algorithms exhibited the shortest inference time for both data sets (0.47 milliseconds for both in the mass spectrometry data set 0.39 and 0.47 milliseconds, respectively, for the urinalysis data set). The experimental results indicated that the RF and XGB algorithms achieved the two highest AUROC values for both data sets (84.7% and 83.9%, respectively, for the mass spectrometry data set 91.1% and 91.4%, respectively, for the urinalysis data set).
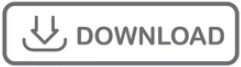